Taking Data in Manufacturing to a Higher Level
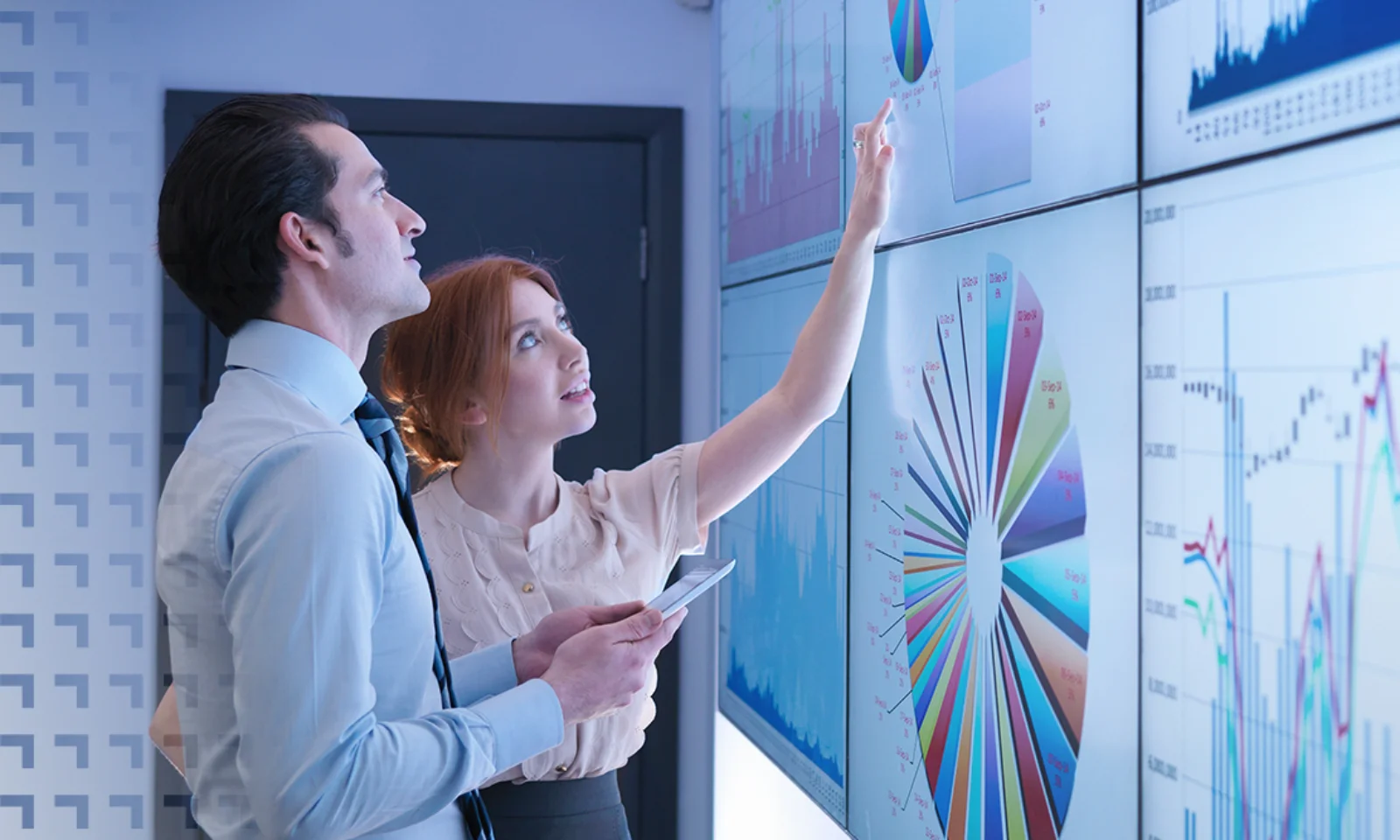
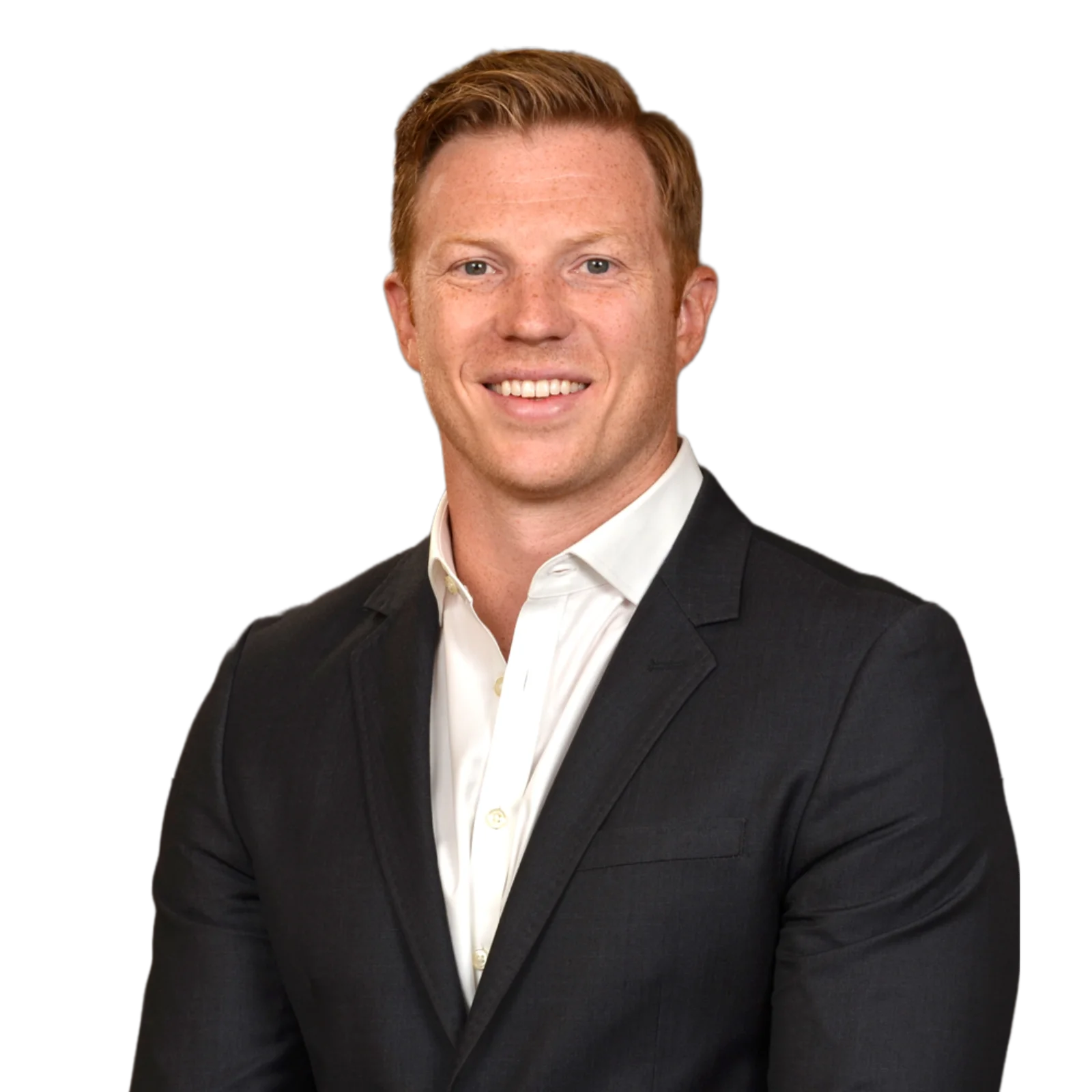
GFT US
Manufacturing experts at a GFT dinner in New York City see generative AI changing how factory data will be used to improve efficiency and boost returns.
Business leaders always find the newest technologies alluring. As the world has become captivated with generative artificial intelligence (Gen AI), they have naturally begun to ask how it can be used across the enterprise—including in manufacturing.
The potential for generative AI tools to wring greater benefits and higher returns from factory automation was certainly front-of-mind among the manufacturing data experts GFT gathered at a dinner at the University Club in New York City in April. But these executives and managers also offered lessons from their use of data and automation today.
Indeed, the experiences shared at the dinner suggested that every advance in the use of factory data, whether it’s cutting edge AI or more mature technologies, is likely to turn on three key questions: What data are you collecting? Where does the data land? And how do you expect to use the data in the future? Taken together, these topics form a framework that can benefit every enterprise that is seeking more effective and profitable use of manufacturing data.
Here’s some of what we heard on each of these three issues:
Data collection. Attendees said the manufacturing equipment they have in place today mostly can provide the data they want. Additional sensors may be needed for specific purposes, but modern equipment and systems typically capture significantly more data than is actually being used.
Data organization. Bigger hurdles are to be found in the effort to ensure large data sets are structured and stored so they can be used for higher level applications. Factory data needs to flow into a system with a modern data architecture. Several guests at the dinner conceded that their companies struggle to get raw data into useful formats. Increasingly, the cloud is the answer, allowing for scalability and resource efficiency while also enforcing consistency and standardization.
Use cases. To enable more sophisticated applications that make use of their factory data, organizations need to know what’s possible, see how that intersects with business priorities, and then chart the path to execution. Some use cases have gained wide acceptance, such as computer vision for quality control. Based on the dinner conversation, however, there’s a shared belief that more—perhaps much more—is necessary and possible.
Interfacing with the data
Improvements in digital manufacturing will always depend on the interface that allows the business to interact with the data it collects and saves. The interface, typically made up of dashboards and charts and graphs, needs to be meaningful for the goals of the business.
As described by one of our dinner guests, an executive from a global manufacturer of heating and cooling equipment and other products, the organization’s data interfaces need regular attention. As requirements change and as capabilities become more advanced, dashboards need to be improved, redesigned, and in some cases rethought entirely. This is an ongoing challenge for this leader’s manufacturing operations.
At the same time, how people interact with their data may be poised for a dramatic change using generative AI technology. It is already possible to create a manufacturing data interface based on natural language queries, using technology that feels like an AI chatbot—but is tailored to the data and requirements of specific manufacturing business use cases.
This development appears likely to reinforce the need for a robust manufacturing data framework. Dinner guests suggested that, as organizations begin to pursue generative AI tools, the careful collection and curation of large data sets, and their preservation in systems with proper architecture to facilitate access, will only become more important.
Reactive or proactive
One dinner participant, who oversees facilities in multiple locations for a maker of cosmetics and perfumes, said computer vision to detect anomalies or flaws in products during their manufacture and packaging is in use across the organization. Quality control is vitally important for a luxury goods maker, and this executive said computer vision technology performs well, with quantifiable return on investment.
And yet, the next step, using the same factory data to address root causes of product flaws and improve manufacturing efficiency, has been hard to achieve. Reactive use of computer vision data is proven, but proactive use for factory optimization remains elusive.
This company’s experience may be indicative of how far factory automation and digitalization have come—but also where they are stalled. A fuller understanding is needed of how manufacturing data can be used in a broader set of use cases. Then, the data sets need to be created, and the applications and technologies brought to bear, to make these initiatives a reality.
Return on investment
No innovation in manufacturing data management is going to be embraced if the benefits can’t be identified and quantified. As one dinner guest put it, the model you use doesn’t matter if you can’t demonstrate impact in dollars. This individual, who is chief data officer for a defense company, is responsible for finding $100 million in increased profitability through better use of manufacturing data.
Coming up with efficiencies on that scale may become more common as generative AI makes creation of applications easier and faster. We’re not there yet, but it’s coming, to judge by the expectations of our dinner participants. What’s also clear is that the same data framework that can benefit an organization today will only become more valuable as new technologies lead to expanded uses and benefits for properly curated manufacturing data.
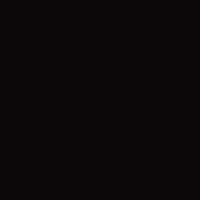