AI data strategy: How to make the most of your data

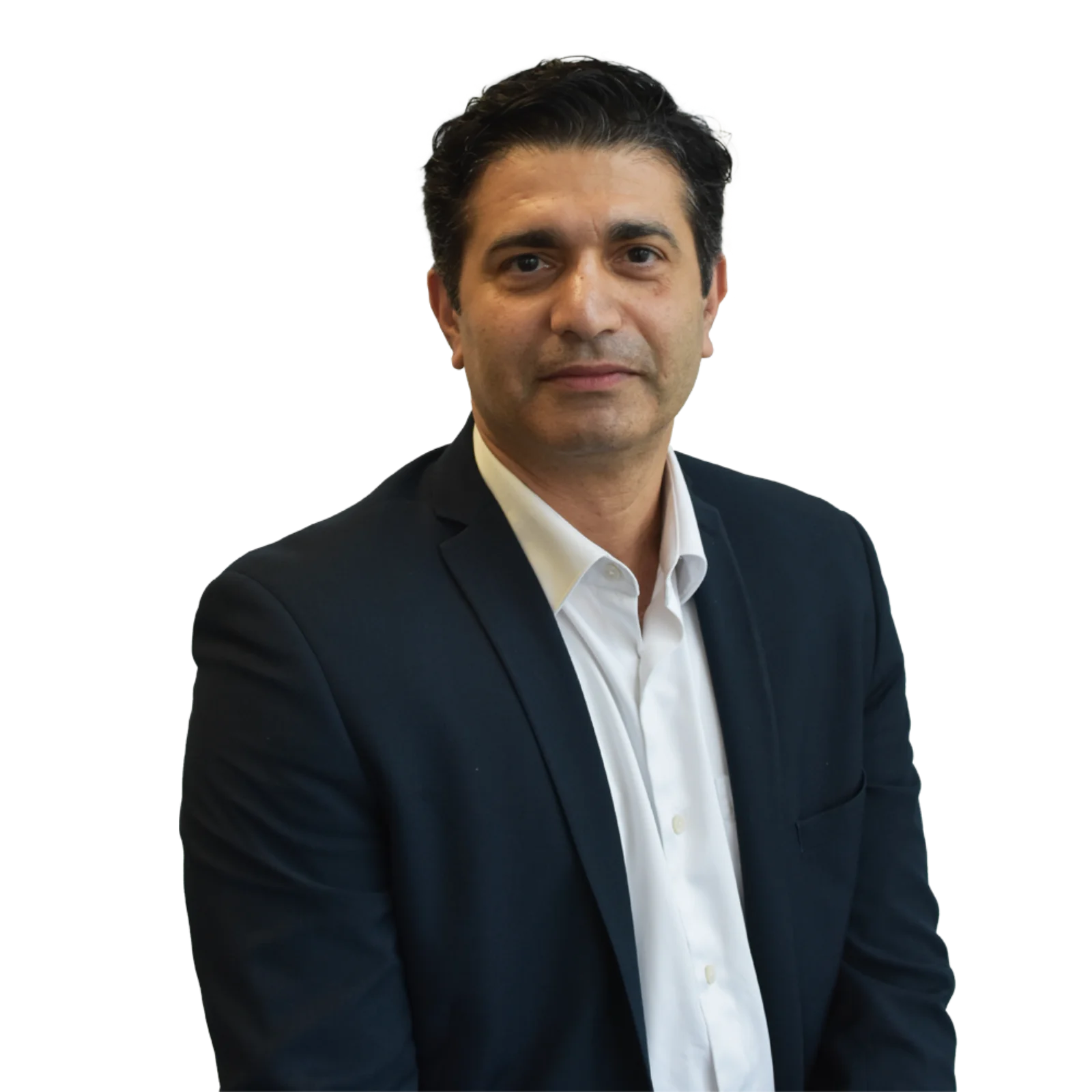
The critical role of data quality
Poor data quality costs organisations an average of $12.9 million annually, according to Gartner www.gartner.com/smarterwithgartner/how-to-improve-your-data-quality. A significant challenge in successfully implementing AI projects arises during the transition from proof of concept (POC) to full-scale deployment. While curating a small dataset for the POC often yields promising results, the real difficulty lies in extending this effort to the broader dataset. This involves comprehensive data cleansing, cataloging, harmonisation and governance - a process where complications and obstacles tend to escalate.
To address these challenges, organisations need to focus on multiple dimensions of data quality management: Data quality encompasses accuracy and consistency across systems, ensuring completeness of datasets with all required elements, maintaining timely updates and availability, confirming relevance to business objectives, and validating compliance with standards. Each of these aspects must be carefully managed to create a solid foundation for AI initiatives.
Building a modern data platform for AI rather than taking a one-size-fits-all approach, successful platforms are built with specific domain requirements in mind.
A modern data platform is a comprehensive system that integrates various data capabilities to address fundamental business challenges such as data redundancy, inconsistent governance, poor distribution and inefficient processing.
The platform architecture typically consists of three fundamental layers:
-
Systems of record for data ingestion and validation
-
Systems of storage and processing for data transformation
-
Systems of engagement for data consumption and analysis
Key components include:
- Data lakes for flexible storage
- Streaming architectures for low latency
- Data warehouses/ lakehouses for structured analytics
- Data fabric to connect them all together
However, simply implementing the technology is not enough - the platform must align with how different business domains actually use and interact with data. Data from various sources also needs to be harmonised; for example, customer data from various systems must be harmonised to create one composite view to reduce duplication and have a common reference to be used across the organisation.
Having a single version of the truth is perhaps most important in the context of master and reference data in modern data platforms.
Optimising data usage: Quality over quantity
While collecting and storing all available data might seem ideal, resource constraints make this approach impractical for most organisations. Instead, a focused strategy targeting the most valuable data sets yields better results. This means being selective about data processing and prioritising quality over quantity.
The key lies in understanding which data sources provide the most business value, how different domains need to access and analyse data, and what level of data quality is required for different applications. This focused approach helps organisations make the most of their limited resources, and yet achieve business successes from their IT initiatives while maintaining high data quality standards.
Our experiences with both external customers and in-house projects consistently prove the value of maintaining a clear focus on data initiatives. By targeting critical datasets, applying precise governance, and ensuring proper adoption, organisations can achieve measurable outcomes while building a scalable, proven methodology for incremental improvement. For example, in a recent project for an asset management service provider, this approach led to a 35% reduction in manual effort, faster insights, and greater agility - showcasing the transformative impact of prioritised, high-quality data initiatives.
Align your data strategy with clear business objectives, prioritising outcomes over technology.
It serves as a straightforward framework of principles and guidelines, enabling organisations to identify their key priorities and align their data efforts and investments to effectively meet those business goals.
This means working closely with stakeholders to identify high-priority use cases and understanding how different business units would actually use the data. The strategy should outline practical steps for improving data quality, implementing governance, and delivering measurable business value.
A successful strategy must be actionable and followed through effectively, avoiding the trap of overthinking and analysis paralysis. This practical mindset enables organisations to transition from planning to tangible implementations.
Ensuring cost-effective implementation
Implementing a comprehensive data strategy requires an investment in technology, processes, and people. GFT recommends several approaches for managing these costs effectively:
-
Start with minimal viable products (MVPs) that deliver quick wins while building toward longer-term goals
-
Focus on domain-specific implementations rather than attempting one-size-fits-all solutions
-
Use a systematic “assess, transform, manage and monetise” (ATMM) approach
-
Prioritise initiatives based on business impact and feasibility
-
Regularly assess ROI to guide course corrections
“We bring strategy to life. By co-creating a working product, you have something you can touch and feel, interrogate and play with. The theory can no start to make sense and you can get a better idea of the requirements and constraints you can stretch or change to have a better understanding of the art of the possible, and thus maximise the probability of success. You can demonstrate this to your wider stakeholders, get their feedback and gain buy-in to a real value product rather than mere slides.”
Getting started with GFT
GFT supports organisations in developing and implementing their AI data strategies by drawing on the full range of AI and data expertise and solutions within the AI.DA Marketplace. Our approach includes:
- Data Intelligence Maturity Assessment
Analysing what you need to measure and engineer to ensure business objectives and how to disseminate a data driven culture within the organisation
- AI + Data Workshops
Workshop-based approach to identify AI and Data Intelligence opportunities. Understand how you could test drive the innovations the world is benefitting from in data and AI using the AI.DA Marketplace.
- AI Experience Framework
Design-led, human driven solutions enabled by AI and trusted data
- Strategy and Roadmap Development leading to deployments
Creating prioritised actionable plans based on evidence and business needs which bring the spectrum of stakeholders to a common understanding of how these initiatives would benefit them
There’s no getting away from the fact that success in implementing a worthwhile AI data strategy requires a considerable amount of technical expertise. However, it demands a continually clear understanding of business objectives, cultural alignment, strong stakeholder buy-in, and careful planning to show tangible value effectively. By partnering with GFT, organisations can access the expertise, strategic experience and engineering excellence needed to help transform their data into genuine strategic assets.
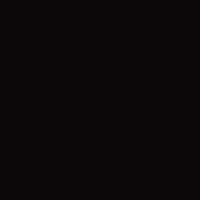
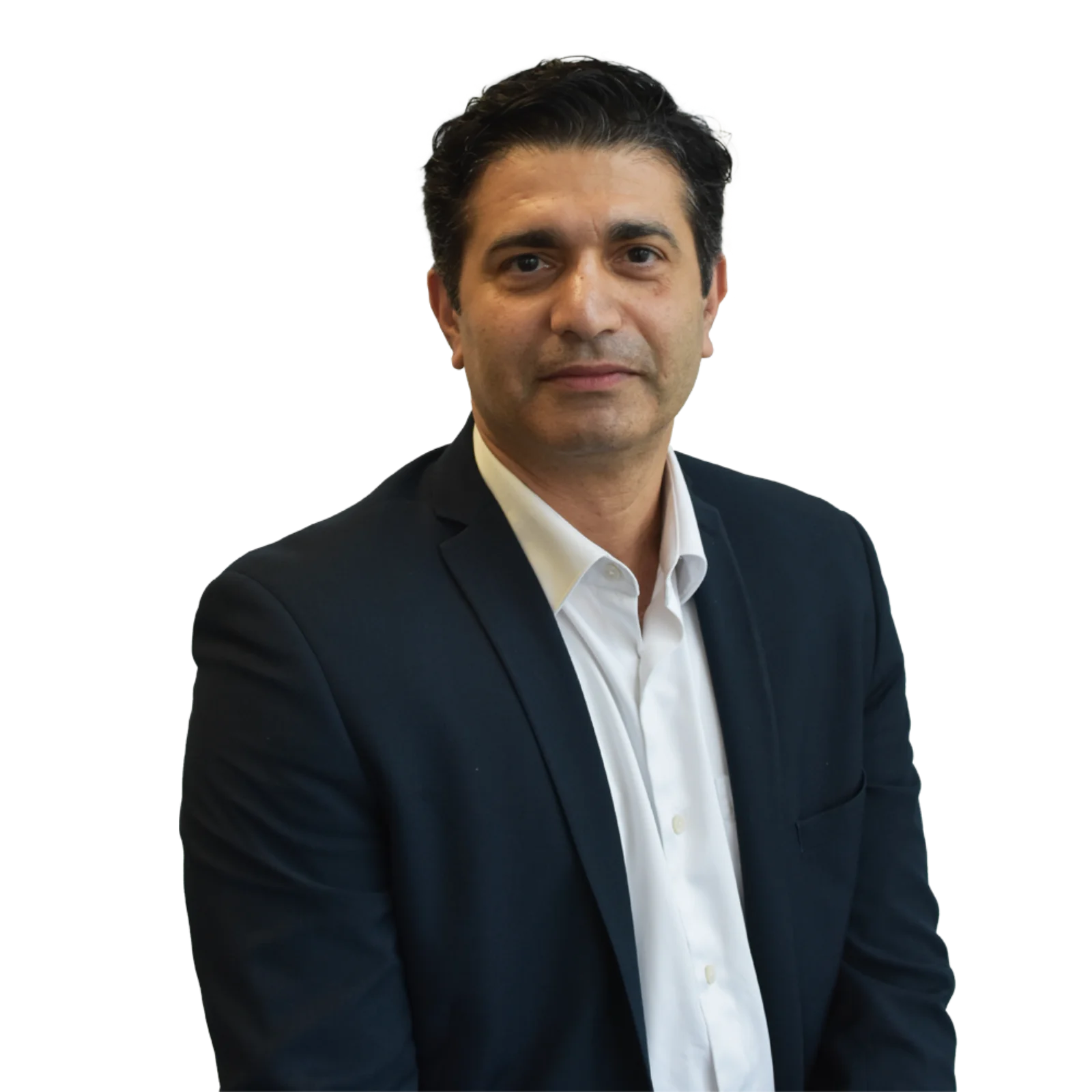